AI Language Learning Mirrors Ancient Human Writing Evolution, New Study Finds
Discover how AI learns languages visually—mirroring ancient writing evolution—by leveraging visual theory of mind, bridging the gap between pictographs and abstract symbols.
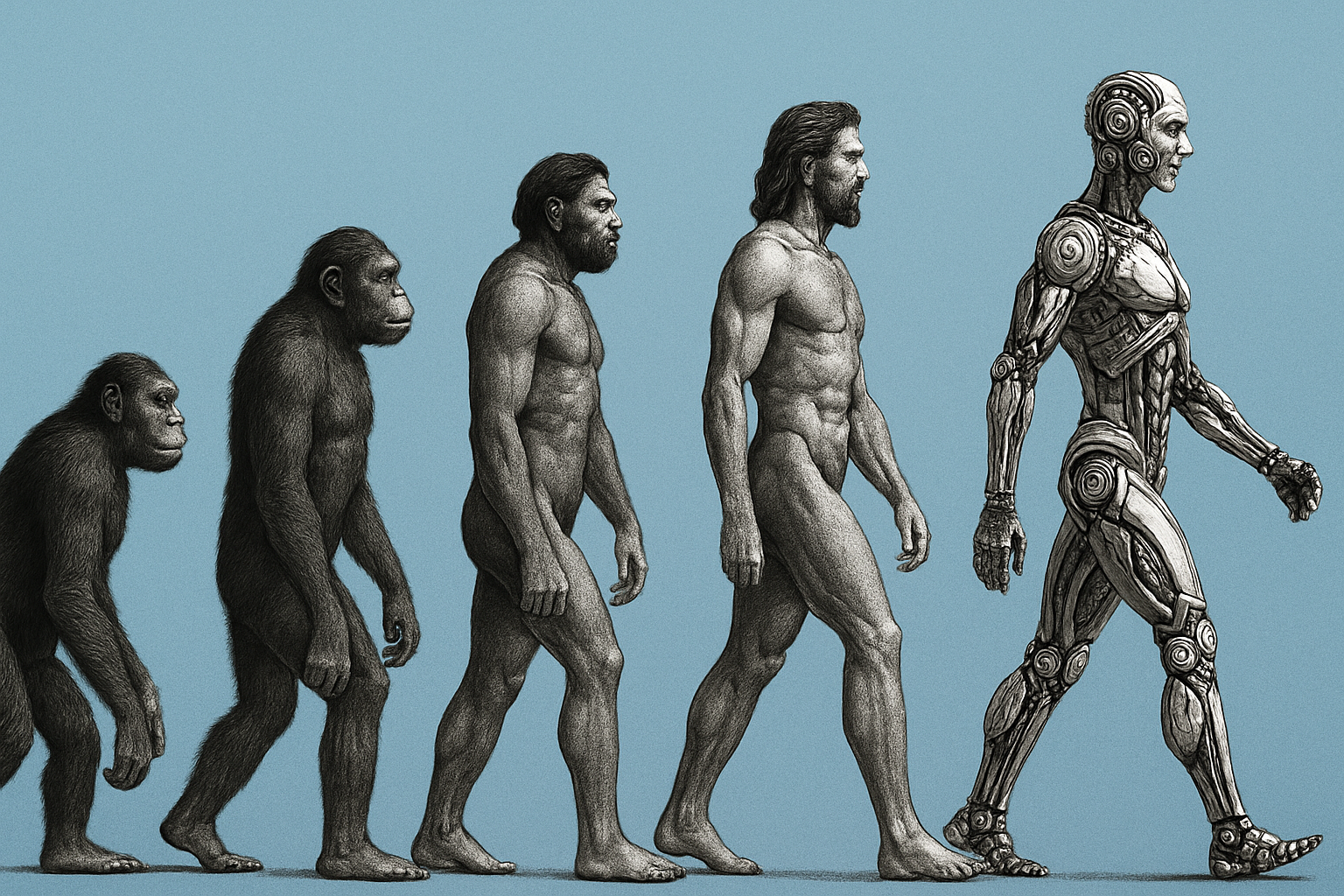
Research from the Cognitive Science Society reveals that artificial intelligence systems learn new languages by processing characters visually—much like how humans developed the earliest writing systems thousands of years ago. This groundbreaking study, led by Benjamin Spiegel, demonstrates that the path from pictographs to abstract symbols follows similar patterns in both AI and human cognitive development.
Visual Processing: The Key to Language Evolution
When learning to recognize and reproduce written language, AI models approach characters as visual patterns rather than abstract symbols with assigned meaning. This process parallels how early humans first developed writing systems based on pictorial representations before evolving toward more abstract symbols.
The research introduces the concept of "visual theory of mind," which refers to understanding how others perceive visual signals. This cognitive ability allows both creators and interpreters of symbols to effectively communicate by leveraging shared visual understanding—a crucial element in both AI learning and human writing system development.
"Visual theory of mind enables agents to reason about how receivers will interpret their drawings," Spiegel explains in the study. "This creates a feedback loop that drives the evolution from iconic pictographs toward more abstract symbolic representations."
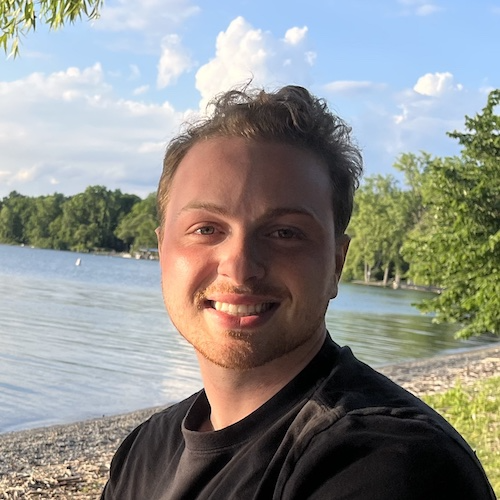
The Signification Game Framework
To investigate this phenomenon, researchers created an innovative multi-agent reinforcement learning environment called the "Signification Game." In this framework, AI agents communicate by creating and interpreting pictorial symbols drawn as splines on a canvas without relying on pre-existing language—a naturalistic approach offering clear parallels to human cognitive development.
Unlike previous computational models based solely on reward-maximization, which the study found severely limited for language acquisition, the Signification Game incorporates inferential communication mechanisms that more accurately reflect how humans develop communication systems.
Bridging the Signification Gap
A critical challenge identified by the researchers is the "signification gap"—the disparity between complex concepts and the limited representational capacity of simple pictographs. To overcome this challenge, both AI and humans employ increasingly sophisticated reasoning about how visual signals will be perceived.
The study demonstrates that as communication needs become more complex, AI agents naturally transition from detailed pictorial representations to more stylized, abstract symbols—precisely mirroring the evolutionary path from ancient Sumerian pictographs to cuneiform script or Egyptian hieroglyphics to hieratic writing.
Implications for AI Development and Cognitive Science
This research provides valuable insights into both artificial and human cognition, suggesting that certain aspects of language development may be universal across different types of intelligence. The findings challenge previous assumptions about language acquisition, demonstrating that visual processing plays a more fundamental role than previously recognized.
"By understanding how AI naturally develops proto-writing systems, we gain insights into the cognitive mechanisms that likely drove human writing evolution," notes Spiegel. "This opens new avenues for developing more human-like AI communication systems while simultaneously illuminating our own cognitive history."
The study represents an important interdisciplinary contribution, bridging artificial intelligence, cognitive science, archaeology, and linguistics to reveal fundamental patterns in how intelligent systems—whether silicon or carbon-based—develop sophisticated communication through visual reasoning.