MAGI-1: Sand AI Disrupts Video Generation with Breakthrough Autoregressive Approach
Sand AI’s open-source MAGI-1 pioneers autoregressive video generation, using chunk-by-chunk processing to achieve unmatched temporal consistency and scalable synthesis through its 24B-parameter Transformer-VAE architecture.
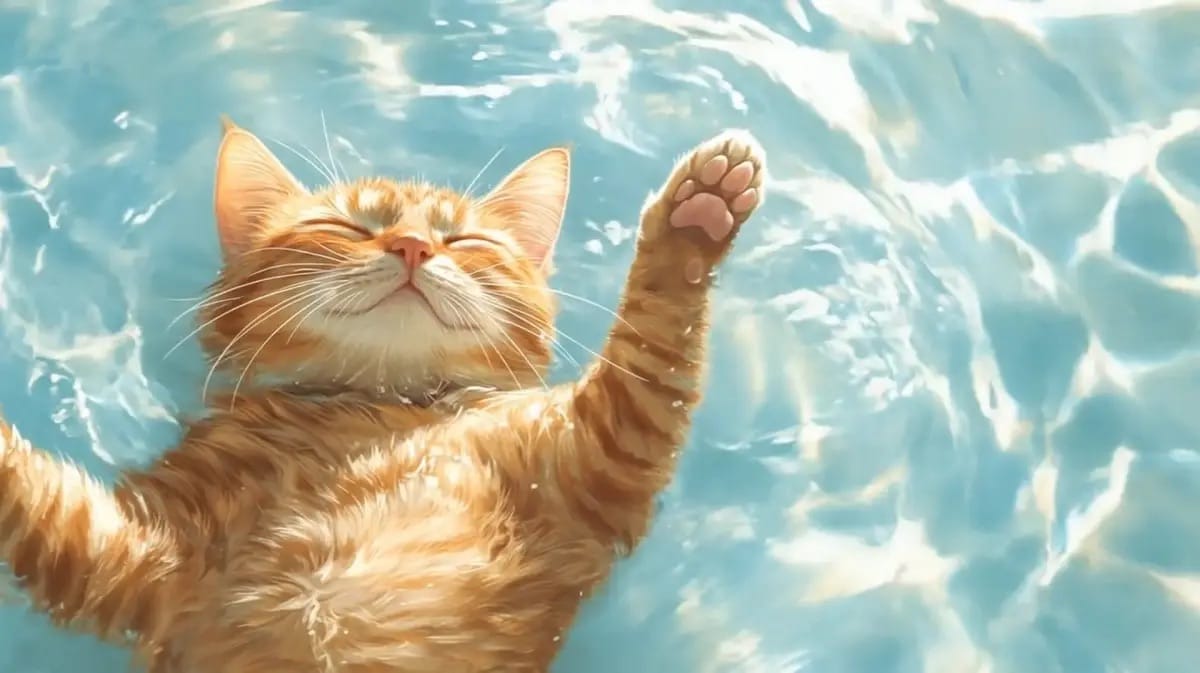
A small AI lab called Sand AI has shaken up the video generation landscape with the release of MAGI-1, the first major video language model (VLM) to leverage autoregressive techniques for creating high-quality videos. Unlike conventional models that generate entire videos at once, MAGI-1 builds videos frame-by-frame, creating each new segment with knowledge of what came before.
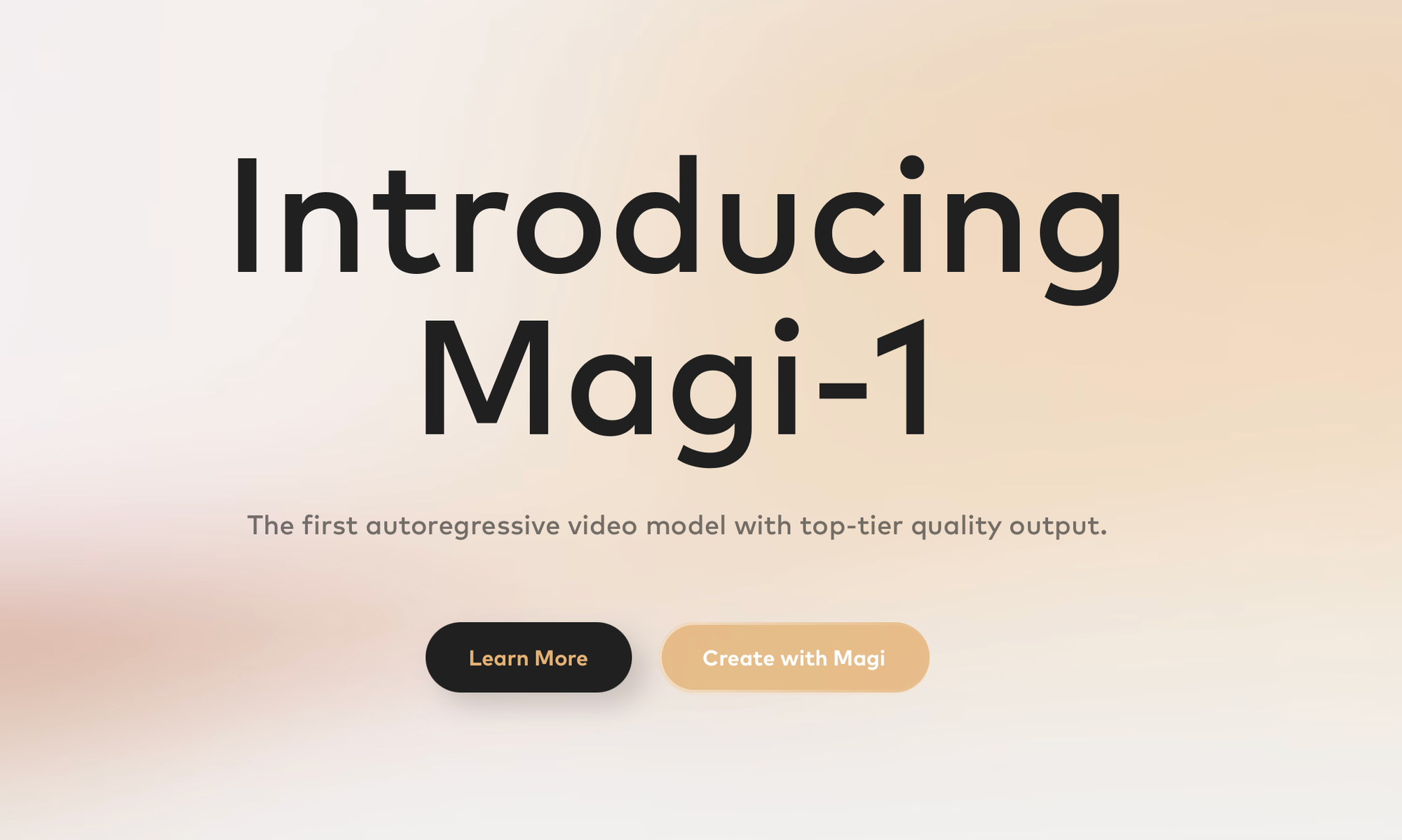
MAGI-1's revolutionary approach addresses a persistent challenge in AI video generation: maintaining consistent characters, scenes, and styles throughout longer clips. By generating 24-frame chunks sequentially and conditioning each new segment on previously created content, the model achieves remarkable temporal consistency while making efficient use of computational resources.
The flagship 24-billion-parameter model incorporates a sophisticated Transformer-based Variational Autoencoder (VAE) architecture that applies 8x spatial and 4x temporal compression to video data. This design choice enables fast decoding while preserving high reconstruction quality—a critical balance in video generation tasks. For users with more limited hardware, Sand AI also offers a 4.5-billion-parameter variant that maintains impressive capabilities while running on a single high-end consumer GPU like the RTX 4090.
Magi-1: The Autoregressive Diffusion Video Generation ModelMAGI-1MAMM
Technical innovation runs throughout MAGI-1's architecture. Block-Causal Attention mechanisms, Parallel Attention Blocks, and specialized normalization techniques work together to enhance training efficiency and stability. The model's advanced attention system processes queries, keys, and values derived from input representations to effectively capture complex spatial-temporal relationships across video frames.
MAGI-1's autoregressive design delivers tangible advantages in benchmark testing. The model achieves a Physical IQ Score of 56.02 in video-to-video tasks, dramatically outperforming competitors like VideoPoet (29.50) and Kling1.6 (23.64). This superior performance stems from the model's ability to build contextual understanding of physical scenes as it generates each new segment.
Perhaps most significantly, MAGI-1's approach solves the fundamental challenge of long-form video generation. Traditional models struggle with the computational demands of processing entire high-resolution videos simultaneously. By breaking this process into manageable chunks, MAGI-1 supports both extended duration videos and controllable generation through chunk-wise prompting, enabling smooth scene transitions and fine-grained text control.
Sand AI's decision to open-source this technology signals a significant milestone in democratizing advanced video generation capabilities. As developers and researchers explore MAGI-1's potential, we're likely to see an explosion of applications that leverage its unique combination of quality, consistency, and scalability across creative and practical use cases.