MIT's "Periodic Table of Machine Learning" Sets Stage for Systematic AI Innovation
MIT’s “periodic table” for machine learning organizes algorithms via shared math principles, enabling systematic AI innovation and improved model performance.
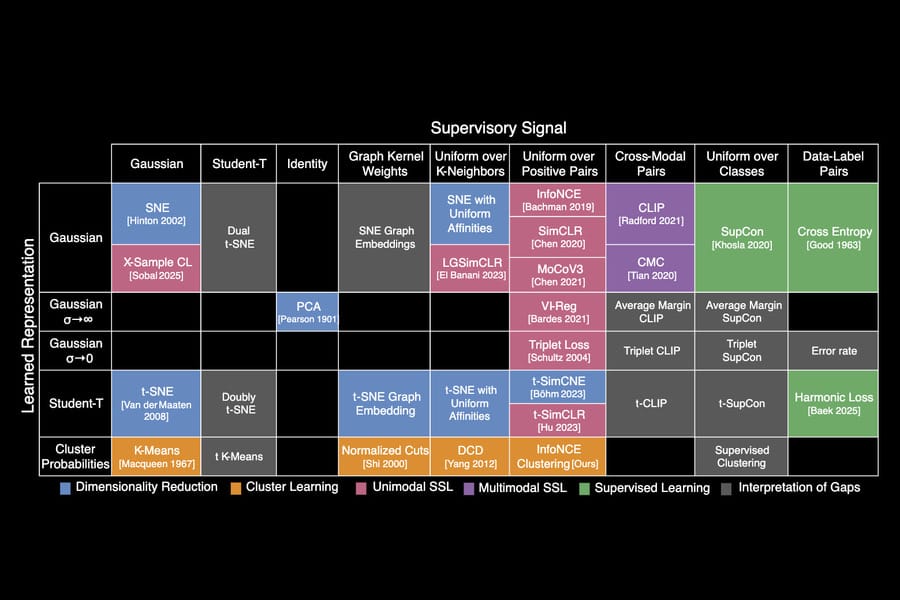
Researchers at the Massachusetts Institute of Technology (MIT) have developed a revolutionary framework that organizes machine learning algorithms in a structure reminiscent of chemistry's periodic table. Called Information Contrastive Learning (I-Con), this organizational system maps relationships between over 20 classical machine learning algorithms through a unifying mathematical foundation.
The framework's power lies in its ability to demonstrate that seemingly different algorithms-from classification and clustering to dimensionality reduction-are fundamentally variations of the same mathematical approach, just optimizing different aspects of data relationships. Like Mendeleev's periodic table that transformed chemistry by organizing elements according to their properties, I-Con arranges algorithms based on how they approximate relationships in data.
"Our framework provides researchers with mathematical tools to design new algorithms without needing to rediscover ideas from prior approaches," explained Shaden Alshammari, lead author of the research. This systematic approach replaces the traditional trial-and-error method of algorithm development with a more structured exploration of the algorithmic landscape.
One of the most intriguing aspects of I-Con is its predictive capability. The table contains empty spaces that theoretically suggest where undiscovered algorithms should exist-similar to how the original periodic table predicted elements before they were discovered. This feature gives AI researchers a roadmap for exploring new algorithmic territory.
The framework has already proven its practical value beyond theoretical organization. MIT researchers demonstrated its utility by combining elements from two different algorithms to create a new image-classification algorithm that performed 8 percent better than existing state-of-the-art methods.
Beyond specific applications, I-Con serves as a navigation tool through the complex landscape of machine learning methods. By visually organizing algorithms and clarifying connections between different approaches, it helps researchers identify patterns and potential combinations that weren't previously obvious.
As machine learning continues advancing at breakneck speed, frameworks like I-Con become increasingly valuable for avoiding redundant research paths while identifying promising new directions. Much like its chemical counterpart revolutionized understanding of elements, this periodic table of algorithms could fundamentally transform how researchers approach AI development, making innovation more systematic and intentional rather than dependent on chance discoveries.
Link: https://news.mit.edu/2025/machine-learning-periodic-table-could-fuel-ai-discovery-0423