Small Language Models: Why Less Might Actually Be More in AI's Next Chapter
Small Language Models (SLMs) provide efficient, specialized AI solutions for healthcare, agriculture, and education, reducing costs and energy use while enabling localized, sustainable applications without massive infrastructure demands.
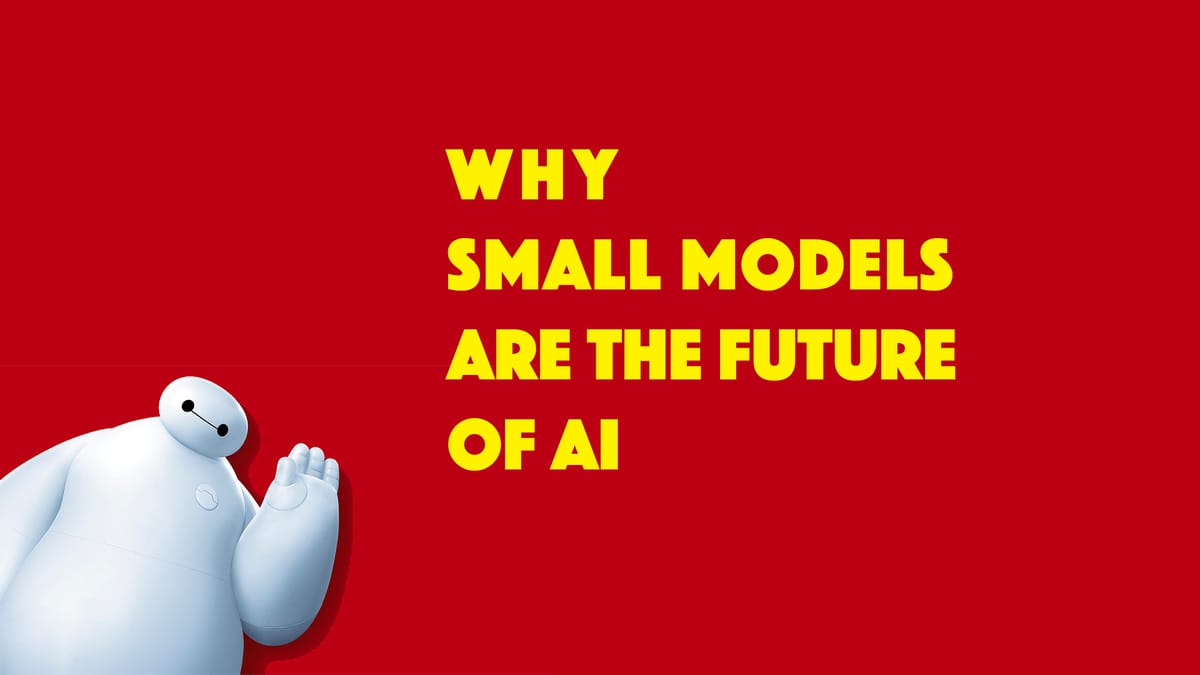
Let’s be honest - when you hear about breakthroughs in artificial intelligence, you’re probably picturing supercomputers crunching numbers in some distant data center. But what if the next big shift in AI isn’t about building bigger systems, but smarter, leaner ones? That’s exactly what’s happening right now with Small Language Models (SLMs), and it’s changing how we think about what AI can do.
I’ve been following the AI space for years, and even I was surprised when researchers started raving about models with under 10 billion parameters. After all, we’ve been conditioned to believe that bigger equals better in tech. But here’s the thing: these compact models aren’t just cheaper to run - they’re proving they can handle real-world tasks without the baggage of their massive counterparts. Let’s unpack why this matters.
The Elephant in the Server Room: Why Bigger Isn’t Always Better
Remember when GPT-3 dropped in 2020? It felt like magic - a model that could write poems, debug code, and even mimic your writing style. But behind the scenes, training that beast required enough energy to power a small town. Google’s Gemini 1.0 Ultra? Rumor has it the training bill alone could’ve funded a mid-sized startup.
Here’s the problem nobody’s talking about: we’re building Ferraris when most people just need reliable commuter cars. Sure, massive models excel at open-ended tasks, but ask yourself - how often do we actually need an AI that can discuss quantum physics and write sonnets about pizza? For most practical applications, it’s overkill.
The environmental angle keeps me up at night. A single LLM training session can emit as much carbon as five cars over their entire lifetimes. And for what? To power chatbots that often struggle with basic customer service queries? There’s got to be a better way.
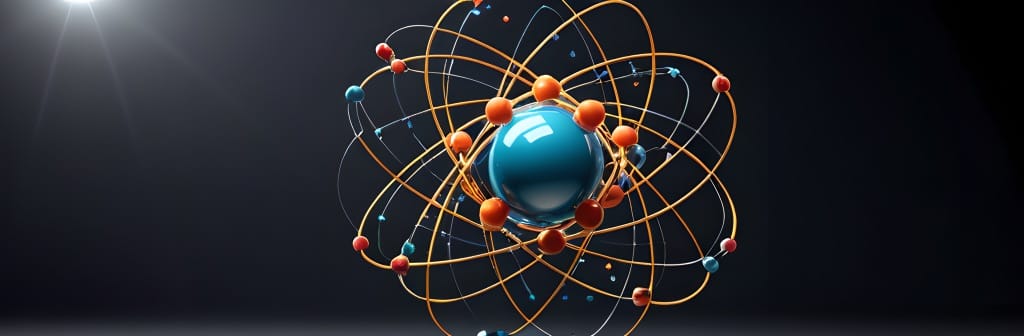
SLMs: The Pocket-Sized Revolution
Enter Small Language Models. These aren’t just scaled-down versions of their bigger siblings - they’re purpose-built tools designed with specific jobs in mind. Think of them as the specialized kitchen gadgets of AI: a garlic press might not do everything a food processor can, but it’s way better at its one job.
Last month, I tested a 3-billion parameter model on my laptop - no internet connection needed. It summarized research papers faster than I could brew coffee. The kicker? It used less power than my desk lamp. This changes everything for:
- Healthcare providers needing real-time analysis of patient data without cloud delays
- Smart home devices that can actually understand context rather than just parsing commands
- Researchers working in field locations with spotty connectivity
But here’s where it gets interesting. These models aren’t just efficient—they’re sneaky good at avoiding the “uncanny valley” effect that makes some AI interactions feel off. Without the bloat of unnecessary parameters, they tend to stay on-task better than models that are trying to be all things to all people.
Teaching Old Models New Tricks
The secret sauce? Training techniques borrowed from how humans learn. Take knowledge distillation—it’s like having a wise mentor (the big model) create focused study guides for their protege (the SLM). Instead of dumping the entire internet into the training data, these distilled datasets cut out the noise.
Then there’s neural pruning. Imagine your brain deciding which neural pathways to keep during development. Researchers are now applying similar principles to AI, systematically trimming redundant connections. The result? Models that run lean without losing their edge. One team at Microsoft recently showed how pruned models actually outperformed larger ones on specific medical diagnostic tasks.
Where Rubber Meets Road: Real-World Wins
Let’s cut through the hype with some concrete examples:
- Agriculture: John Deere’s using SLMs in tractors to analyze soil data on-the-fly, adjusting planting patterns without waiting for cloud processing. Farmers in Iowa are seeing 12% higher yields just from real-time adjustments.
- Education: Duolingo’s new grammar checker for language learners runs entirely on-device. No more privacy concerns about kids’ voice data hitting the cloud.
- Disaster Response: Red Cross field units in earthquake zones are using SLM-powered tablets that work even when cell towers go down. These devices can translate between rescue workers and survivors without internet access.
The pattern’s clear—when you design AI for specific scenarios rather than general prowess, you unlock solutions that are both more effective and more accessible.
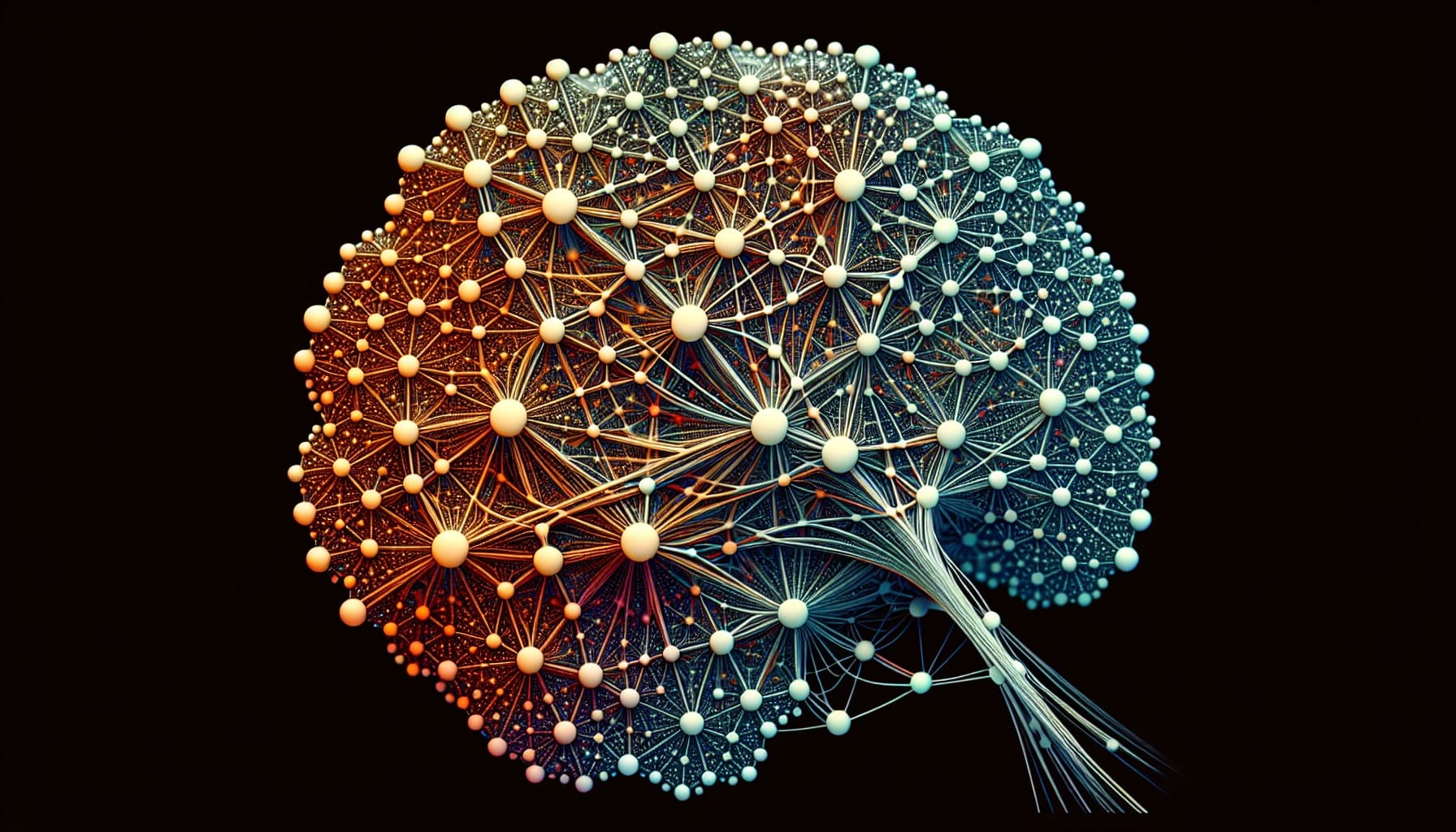
The Road Ahead: Smarter, Not Harder
Does this mean the era of giant AI models is over? Hardly. There’s still a place for behemoths like GPT-4 in research and complex creative tasks. But the landscape is shifting toward a mix-and-match approach.
Companies like Apple and Samsung are betting big on SLMs for their next-gen devices. Rumor has it the iPhone 17 will ship with a dedicated AI chip for on-device SLMs. Meanwhile, open-source communities are exploding with specialized models—there’s now an SLM fine-tuned specifically for explaining physics concepts to middle-schoolers.
What excites me most isn’t just the technology—it’s the democratization. With lower barriers to entry, we’re seeing startups and even university labs create impactful AI tools without needing Bezos-level funding. A student team from MIT recently developed an SLM that helps non-verbal autism patients communicate, trained entirely on a cluster of gaming PCs.
The Bottom Line
We’re at an inflection point. For years, AI progress was measured in parameters and training budgets. Now, the conversation’s shifting to efficiency and practicality. Small Language Models aren’t just a technical curiosity—they’re a necessary evolution toward sustainable, accessible AI that solves real problems rather than just dazzling us with scale.
The next time you hear about a billion-dollar AI project, ask yourself: could a carefully crafted smaller model do the job better? Increasingly, the answer might surprise you. After all, the best tools aren’t always the biggest—they’re the ones that fit perfectly in your hand.